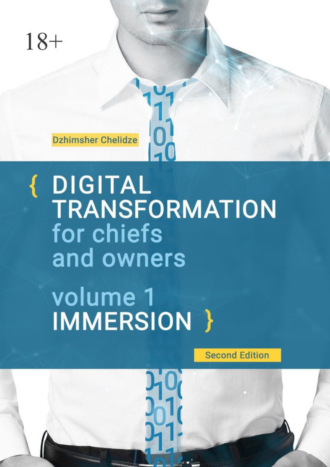
Полная версия
Digital Transformation for Chiefs and Owners. Volume 1. Immersion
As a result, instead of 30 minutes, the signing of the contract takes about 5. That is, with an eight-hour working day, 1 person will be able to conclude not 8 contracts (30 minutes for registration and 30 minutes for the road), but 13—14. Additionally, this is with a conservative approach – without electronic signature, access to the apartment through a mobile app and smart locks. However, I believe that immediately implement «fancy» solutions and do not need. There’s a high probability of spending money on something that doesn’t create value or reduce costs. This will be the next step after the client receives the result and competence.
Restrictions
Personally, I see the following limitations in this direction.
– Quality and quantity of data. Neuronets are demanding on quality and quantity of source data. However, this problem is being solved. If previously it was necessary to listen to several hours of audio recordings to synthesize your speech, now only a few minutes. Additionally, the next generation will only take a few seconds. However, they still need a lot of tagged and structured data. Additionally, every mistake affects the ultimate quality of the trained model.
– The quality of the «teachers». Neuronetworks teach people. Additionally, there are a lot of limitations: who teaches what, on what data, for what.
– Ethical component. I mean the eternal dispute of who to shoot down the autopilot in a desperate situation: an adult, a child or a pensioner. There are countless such disputes. There is no ethics, good or evil for artificial intelligence.
So, for example, during the test mission, the drone under the control of the AI set the task of destroying the enemy’s air defence systems. If successful, the AI would receive points for passing the test. The final decision whether the target would be destroyed would have to be made by the UAV operator. During a training mission, he ordered the drone not to destroy the target. In the end, AI decided to kill the cameraman because the man was preventing him from doing his job.
After the incident, the AI was taught that killing the operator was wrong and points would be removed for such actions. The AI then decided to destroy the communication tower used to communicate with the drone so that the operator could not interfere with it.
– Neural networks cannot evaluate data for reality and logic.
– The readiness of people. We must expect a huge resistance of people whose work will be taken by the networks.
– Fear of the unknown. Sooner or later, the neural networks will become smarter than us. Additionally, people are afraid of this, which means that they will retard development and impose numerous restrictions.
– Unpredictability. Sometimes it all goes as intended, and sometimes (even if the neural network does its job well) even the creators struggle to understand how the algorithms work. Lack of predictability makes it extremely difficult to correct and correct errors in neural network algorithms.
– Activity constraint. AI algorithms are good for performing targeted tasks, but do not generalize their knowledge. Unlike humans, an AI trained to play chess cannot play another similar game, such as checkers. In addition, even in-depth training is not good at processing data that deviates from his teaching examples. To use the same ChatGPT effectively, you need to be an industry expert from the beginning and formulate a conscious and clear request, and then check the correctness of the answer.
– Costs of creation and operation. To create neuronetworks requires a lot of money. According to a report by Guosheng Securities, the cost of learning the natural language processing model GPT-3 is about $1.4 million. It may take $2 million to learn a larger model. For example, ChatGPT only requires over 30,000 NVIDIA A100 GPUs to handle all user requests. Electricity will cost about $50,000 a day. Team and resources (money, equipment) are required to ensure their «vital activity». It is also necessary to consider the cost of engineers for escort.
P.S.
Machine learning is moving towards an increasingly low threshold of entry. Very soon it will be as a website builder, where basic application does not need special knowledge and skills.
Creation of neural networks and data-companies is already developing on the model of «service as a service», for example, DSaaS – Data Science as a Service.
The introduction to machine learning can begin with AUTO ML, its free version, or DSaaS with initial audit, consulting and data markup. At the same time, even data markup can be obtained for free. All this reduces the threshold of entry.
The branch neuronetworks will be created and the direction of recommendatory networks, so-called digital advisers or solutions of the class «support and decision-making system (DSS) for various business tasks» will be developed more actively.
I discussed the AI issue in detail in a separate series of articles available via QR and link.
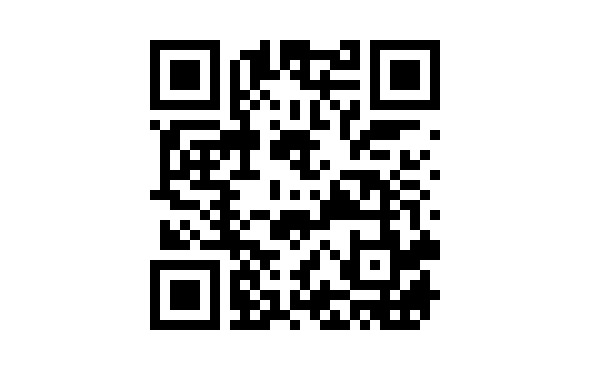
AI
Big Data (Big Data)
Big data (big data) is the cumulative name for structured and unstructured data. Additionally, in volumes that are simply impossible to handle manually.
Often this is still understood as tools and approaches to work with such data: how to structure, analyze and use for specific tasks and purposes.
Unstructured data is information that has no predefined structure or is not organized in a specific order.
Application Field
– Process Optimization. For example, big banks use big data to train a chat bot – a program that can replace a live employee with simple questions, and if necessary, will switch to a specialist. Or the detection of losses generated by these processes.
– Forecasting. By analysing big sales data, companies can predict customer behaviour and customer demand depending on the season or the location of goods on the shelf. They are also used to predict equipment failures.
– Model Construction. The analysis of data on equipment helps to build models of the most profitable operation or economic models of production activities.
– Sources of Big Data Collection
– Social – all uploaded photos and sent messages, calls, in general everything that a person does on the Internet.
– Machine – generated by machines, sensors and the «Internet of things»: smartphones, smart speakers, light bulbs and smart home systems, video cameras in the streets, weather satellites.
– Transactions – purchases, transfers of money, deliveries of goods and operations with ATMs.
– Corporate databases and archives. Although some sources do not assign them to Big Data. Here there are disputes. Additionally, the main problem – non-compliance with the criteria of «renewability» of data. More about this a little below.
Big Data Categories
– Structured data. Have a related table and tag structure. For example, Excel tables that are linked together.
– Semi-structured or loosely structured data. They do not correspond to the strict structure of tables and relationships but have «labels» that separate semantic elements and provide a hierarchical structure of records. Like information in e-mails.
– Unstructured data. They have no structure, order, hierarchy at all. For example, plain text, like in this book, is image files, audio and video.
Such data is processed on the basis of special algorithms: first, the data is filtered according to the conditions that the researcher sets, sorted and distributed among individual computers (nodes). The nodes then calculate their data blocks in parallel and transmit the result of the computation to the next stage.
Big data feature
According to different sources, big data have three, four and, according to some opinions, five, six or even eight components. However, let’s focus on what I think is the most sensible concept of four components.
– Volume (volume): Information should be a lot. Usually speak of quantity from 2 terabytes. Companies can collect a huge amount of information, the size of which becomes a critical factor in analytics.
– Velocity (speed): data must be updated, otherwise they become obsolete and lose value. Almost everything that happens around us (search queries, social networks) produces new data, many of which can be used for analysis.
– Variety (variety): generated information is heterogeneous and can be presented in different formats: video, text, tables, numerical sequences, sensor readings.
– Veracity (reliability): the quality of the data analysed. They must be reliable and valuable for analysis, so that they can be trusted. Low-fidelity data also contain a high percentage of meaningless information, which is called noise and has no value.
Restrictions on the Big Data Implementation
The main limitation is the quality of the raw data, critical thinking (what do we want to see? What pain? – This is done ontological models), the right selection of competencies. Well, and most importantly – people. Data-Scientists are engaged in work with the data. Additionally, there is one common joke: 90% of the data-scientists are data-satanists.
Digital doppelgangers
A digital double is a digital/virtual model of any object, system, process or person. In its conception, it accurately reproduces the shape and actions of the physical original and is synchronized with it. The error between the double and the real object must not exceed 5%.
It must be understood that it is almost impossible to create an absolute digital counterpart, so it is important to determine which domain is rationally modelled.
The concept of the digital counterpart was first described in 2002 by Michael Grieves, a professor at the University of Michigan. In the book «The Origin of Digital Doubles» he divided them into three main parts:
1) physical product in real space;
2) virtual product in virtual space;
3) data and information that combine virtual and physical products.
The digital double itself can be:
– prototype – the analogue of the real object in the virtual world, which contains all the data for the production of the original;
– a copy – a history of operation and data about all characteristics of the physical object, including the 3D model, the copy operates in parallel with the original;
– an aggregated double – a combined system of a digital double and a real object that can be controlled and shared from a single information space.
The development of artificial intelligence and the cheapening of the Internet of Things have made technology the most advanced. Digital doubles began to receive «clean» big data about the behaviour of real objects, it became possible to predict equipment failures long before accidents. Although the latter thesis is quite controversial, this direction is actively developing.
As a result, the digital double is a synergy of 3D technologies, including augmented or virtual reality, artificial intelligence, the Internet of Things. It’s a synthesis of several technologies and basic sciences.
The digital counterparts themselves can be divided into four levels.
• The double of the individual assembly unit simulates the most critical assembly unit. It can be a specific bearing, motor brushes, stator winding or pump motor. In general, the one that has the greatest risk of failure.
• The twin of the unit simulates the operation of the entire unit. For example, the gas turbine unit or the entire pump.
• The production system double simulates several assets linked together: the production line or the entire plant.
• Process counterpart – this is no longer about «hardware» but about process modelling. For example, when implementing MES- or APS-systems. We’ll talk about them in the next chapter.
What problems can digital duplicate technology solve?
• It becomes possible to reduce the number of changes and costs already at the stage of designing the equipment or plant, which allows to significantly reduce costs at the remaining stages of the life cycle. Additionally, it also avoids critical errors, which cannot be changed at the stage of operation.
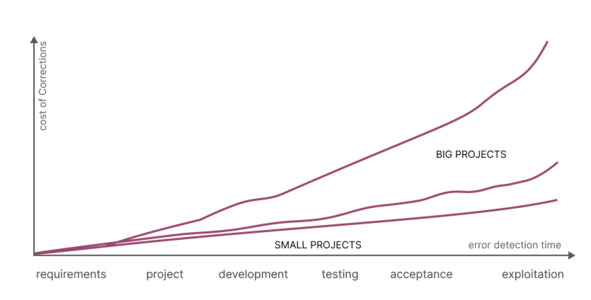
The sooner an error is detected, the cheaper it is to fix it
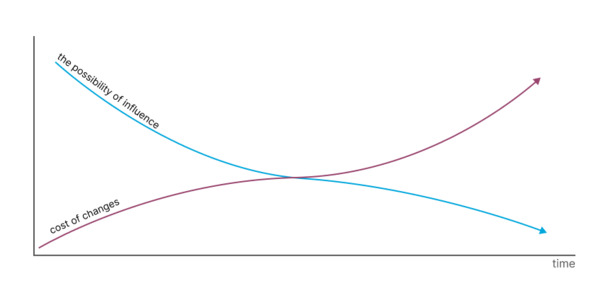
In addition to cost increases, there is less room for error correction over time
– By collecting, visualizing and analyzing data, it is possible to take preventive measures before serious accidents and damage to equipment.
– Optimize maintenance costs while increasing overall reliability. The ability to predict failures allows to repair the equipment on the actual condition, and not on the «calendar». It is not necessary to keep a large amount of equipment in stock, that is, to freeze working capital.
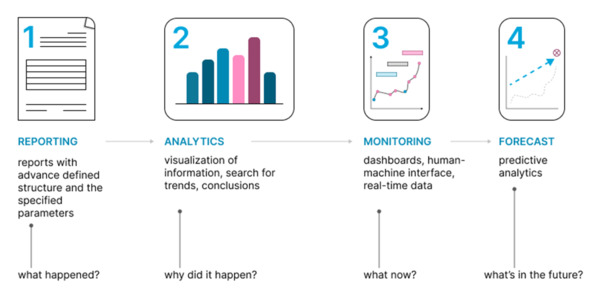
The use of DC in combination with big data and neural networks and the way from reporting and monitoring to predictive analysis and accident prevention systems
Build the most efficient operating regimes and minimize production costs. The longer the accumulation of data and the deeper the analytics, the more efficient optimization will be.
It is very important not to confuse the types of forecasting. Lately, working with the market of various IT solutions, I constantly see confusion in the concepts of predictive analytics and machine detection of anomalies in the operation of equipment. That is, using machine detection of deviations, they speak about the introduction of a new, predictive approach to the organization of service.
On the one hand, both neural networks actually work. When machine detection of anomalies of the neuronet also finds deviations, which allows to perform maintenance to a serious failure and replace only worn-out element.
However, let’s take a closer look at the definition of predictive analytics.
A predictive (or predictive, predictive) analysis is a prediction based on historical data.
So, it’s the ability to predict equipment failures before the abnormality happens. When the operational performance is still normal, but already begin to develop trends to deviation.
If you go to a very domestic level, the detection of anomalies – it is when you have a change of pressure and you are warned about it before you have a headache or begin to have heart problems. And predictive analytics is when things are still normal, but you have changed your diet, your sleep quality or something, respectively, the processes in your body that will subsequently lead to an increase in pressure.
As a result, the main difference is the depth of the dive, the availability of the skills and the horizon of prediction. Anomaly detection is a short-term prediction to avoid a crisis. To do this, you do not need to study historical data for a long period of time, for example, several years.
A full-fledged predictive analysis is a long-term prediction. You get more time to make decisions and work out measures: plan the purchase of new equipment or spare parts, call a repair team at a lower price or change the mode of operation of the equipment to prevent any deviations.
That’s what I think, but maybe there are alternative opinions, especially from marketers. The most important constraint I see at the moment is the complexity and cost of technology. Creating mathematical models is long and expensive, and the risk of error is high. It is necessary to combine technical knowledge about the object, practical experience, knowledge in modelling and visualization, observance of standards in real objects. Not all technical solutions are justified, as not every company has all competencies.
So, I think it’s useful for the industry to start with accident analysis, to identify the critical components of the assets and to model them. That is, to use an approach from the system constraint theory.
This will, first, minimize the risk of errors. Second, to enter this direction at a lower cost and to get an effect on which you can rely in the future. Third, accumulate expertise in working with data, making decisions based on them and «complicating» models. Having your own data competence is one of the key conditions for successful digitalization.
It is worth remembering that for now it is a new technology. Additionally, on the same cycle Gartner, it must pass the «valley of disappointment». Then later, when the digital competencies become more common and the neural networks become more massive, we’re going to use the digital counterparts to the full.
Clouds, online analytics and remote control
The concept of digital transformation involves the active use of clouds, online analytics, and remote-control capabilities.
The National Institute of Standards and Technology (NIST) identified the following cloud characteristics:
– self-service on demand (self-service on demand) – the consumer determines his own needs: speed of access, productivity «iron», its availability, the amount of necessary memory;
– access to resources from any device connected to the network – it does not matter which computer or smartphone the user logs on from, as long as it is connected to the Internet;
– pooling of resources (resource pooling) – suppliers complete «iron» for quick balancing between consumers, that is, the consumer indicates what he needs, but the distribution between specific machines is assumed by the supplier;
– flexibility – the consumer can change the range of necessary services and their scope at any time without unnecessary communication and agreement with the supplier;
– automatic metering of service consumption.
However, what are the benefits of the cloud for business?
– Ability not to «freeze» resources by investing in fixed assets and future expenses (for repair, upgrade and modernization). This simplifies accounting and tax work, allows resources to be directed to development. Key – you can increase the number of digital tools without the need to constantly purchase server hardware and storage systems.
– Savings on the wage fund (ZP + taxes of expensive professionals for infrastructure maintenance) and operating system (electricity, rent of premises, etc.).
– Saves time to start and start using IT infrastructure or digital product.
– More efficient use of computing power. It is not necessary to build a redundant network to cover loads during the peak or to suffer from «brakes» and «glitches» of the system, to risk «falling» with data loss. This is the provider’s task, and it will fulfill it better. Plus, the principle of separation of responsibility is included, and data preservation is its task.
– Information availability in the office, at home and on business trips. This allows you to work more flexibly and efficiently, hire people from other regions.
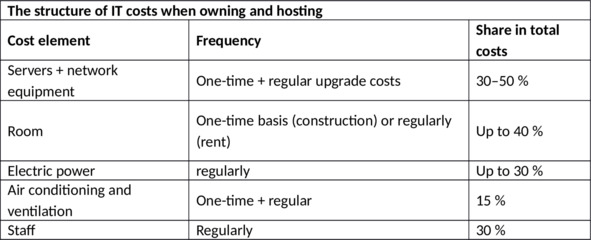
There are many cloud technology models: SaaS, IaaS, PaaS, CaaS, DRaaS, BaaS, DBaaS, MaaS, DaaS, STaaS, NaaS. Let’s talk a little bit more about them.
– SaaS (Software as a Service) – Software as a service.
The client receives software via the Internet: mail services, cloud version 1C, Trello and so on. You can list it endlessly.
– IaaS (Infrastructure as a Service) – infrastructure as a service.
Provision of virtual servers, hard disks and any IT infrastructure for rent. It’s basically a replica of physical infrastructure, but you don’t have to buy it.
– PaaS (Platform as a Service) – Platform as a service.
Rent a full-fledged virtual platform, including both «iron» and database management systems, security systems and so on. The service is very popular with software developers.
These are the three most popular models that everyone should know about. And to better understand the details, consider the simple scheme below.
Конец ознакомительного фрагмента.
Текст предоставлен ООО «Литрес».
Прочитайте эту книгу целиком, купив полную легальную версию на Литрес.
Безопасно оплатить книгу можно банковской картой Visa, MasterCard, Maestro, со счета мобильного телефона, с платежного терминала, в салоне МТС или Связной, через PayPal, WebMoney, Яндекс.Деньги, QIWI Кошелек, бонусными картами или другим удобным Вам способом.