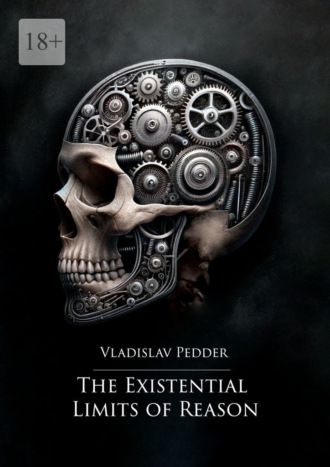
Полная версия
The Existential Limits of Reason
One vivid example of such a phenomenon can be symbiosis – a close, mutually beneficial coexistence of different species. When two or more species cooperate with each other, their chances of survival increase, and their traits can be supported and strengthened through evolutionary mechanisms. In this way, traits like the green beard, over the long term, can spread not only at the level of individual organisms but also within more complex biological systems, contributing to the overall survival of the group.
Today, it is believed that selection occurs on several levels:
Genetic level: Selection occurs at the level of individual genes. Genes that promote the successful survival and reproduction of their carriers become established in the population, passed down to future generations. This selection focuses on how specific genetic variations can increase their frequency in the population through their impact on the organism or on their copies in other organisms.
Individual level: Selection acts at the level of organisms. Individuals with traits that increase their chances of survival and successful reproduction are able to pass their genes to the next generation. This leads to the spread of beneficial adaptations within the population and the establishment of traits that enhance individual fitness.
Kin selection: Selection occurs through helping close relatives who share similar genes. Altruistic behavior toward kin can increase the chances of spreading common genes, even if it reduces individual survival chances. This type of selection explains the emergence of cooperative behavior in family groups and colonies.
Group level: Selection occurs at the level of groups of organisms. Groups in which members cooperate and support each other may have an advantage over groups where selfish behavior predominates. Competition between such groups may lead to the selection of cooperative strategies that enhance the success of the group as a whole.
Ecosystem or symbiotic community level: Selection may occur at the level of entire ecosystems or communities made up of interconnected species. In such systems, stable interactions, such as symbiosis, cooperation, and mutual support, can contribute to the successful existence of all members of the community. If an ecosystem or symbiotic community successfully adapts to changes in the environment and maintains its stability, it can contribute to the survival and spread of all the species involved. Although this level of selection is debated, examples of coevolution show that complex communities can form through cooperative and mutually beneficial relationships between different organisms.
Modern research supports the ideas of multilevel selection, showing how cooperation at the group and community levels can contribute to evolutionary success.
2.5 The Role of Randomness and Directionality in Evolution
It is important to note that evolution, as a process, largely depends on random mutations, which can either benefit or harm an organism. However, the presence of directionality in evolution is not entirely excluded. With each generation, species become more adapted to their environment, but this does not occur through predefined goals or projects. Instead, it is the result of interactions between random changes and prevailing ecological and social factors.
Evolution does not have a predetermined goal or final destination. An important point is that it is not aimed at creating perfect beings but simply at adapting organisms to the specific conditions in which they exist. In this sense, evolution is not so much a process of development as it is one of endless adaptations and changes.
Conclusion
Thus, evolution has no predetermined goal or inherent meaning. Life and death are part of a continuous cycle of changes and adaptations that ensure the survival of species best suited to their environment. Death, as part of this process, does not imply an afterlife; rather, it is necessary for more adapted organisms to continue their existence. Evolution is a sequence of random processes that have ultimately led to the emergence of modern species, including humans. We exist as we are solely because all other variations did not survive, and we do not see them. All life on Earth, from microorganisms to humans, is the result of deterministic processes that, over billions of years, have shaped living beings capable of reproduction and adaptation.
3. The Emergence of Intelligence
Intelligence is one of the most complex achievements of evolution, becoming a key factor in the success of many species, especially humans. In this section, we will explore how evolution led to the emergence of intelligence, examine differences in cognitive development between mammals and cephalopods, and analyze how the brain utilizes predictive coding and Bayesian approaches to process information.
The Emergence of Intelligence: Evolutionary Preconditions
The evolution of intelligence is a gradual process involving the development of increasingly complex cognitive abilities such as learning, memory, prediction, and self-reflection. Intelligence did not arise suddenly; its emergence was the result of millions of years of adaptation to changing environmental conditions.
The most significant steps toward intelligence include:
Development of sensory systems and memory. Organisms began accumulating information about their environment and using it for survival.
Emergence of associative learning. The ability to link stimuli with responses helped in predicting dangers and opportunities.
Development of spatial reasoning. Animals started forming mental representations of their surroundings and planning their actions.
Social interaction. Group interactions facilitated the development of communication and more complex behavioral strategies.
Over time, these elements evolved into advanced cognitive systems capable of abstract thinking, self-awareness, and future planning.
Differences in the Evolution of Intelligence in Mammals and Cephalopods
An intriguing example of the evolution of intelligence can be seen in mammals and cephalopods (such as octopuses) – two distinct evolutionary paths leading to advanced cognition.
Mammals, including humans, developed intelligence in a social context, where cooperation and group living played a crucial role. Their cognitive abilities evolved to solve problems related to cooperation, competition, and social communication. This led to the emergence of complex social hierarchies, empathy, theory of mind (understanding the thoughts and intentions of others), language, and abstract thinking. The mammalian brain features a large cerebral cortex, particularly the frontal lobes, responsible for planning, self-control, and decision-making.
Cephalopods, on the other hand, evolved intelligence in a solitary existence, requiring adaptation to diverse oceanic environments. Their cognitive abilities focus on solving spatial problems, camouflage, tactical behavior, and independent control of limbs. A unique feature of cephalopod brains is that about two-thirds of their neurons are located in their tentacles, allowing their limbs to act autonomously.
These two examples demonstrate that intelligence can evolve through different pathways, adapting to specific survival challenges.
As we continue exploring the evolution of intelligence, understanding how the brain functions and has developed over time remains essential..
The Principle of Brain Functioning
The brain consists of billions of neurons that process information and coordinate the organism’s actions. These neurons communicate with each other through chemical substances called neurotransmitters. When a neuron is activated, it transmits an electrical impulse that reaches the synapse – the contact point with another neuron. At this point, the electrical signal is converted into a chemical one, as neurotransmitters are released into the synaptic cleft and activate receptors on the next neuron.
Key neurotransmitters such as dopamine, serotonin, and glutamate regulate essential aspects of behavior and perception. For example, dopamine is associated with motivation and the reward system, while serotonin influences mood and anxiety levels. Glutamate serves as the primary excitatory neurotransmitter, playing a crucial role in learning and memory processes.
The Influence of Hormones on Brain Function
Hormones play a crucial role in regulating behavior and physiological states. For example, cortisol, the stress hormone, is produced in response to threats and helps the body cope with emergency situations. However, if its levels remain elevated for prolonged periods, it can lead to chronic stress, depression, and impaired cognitive function. Oxytocin, on the other hand, promotes the strengthening of social bonds and empathy, which are essential for complex forms of communication and interaction.
The influence of hormones on the brain is regulated through the hypothalamus, which controls the pituitary gland and, in turn, interacts with the endocrine system. This integration ensures the coordination of cognitive and physiological processes.
The Microbiota and Its Influence on the Brain
The microbiota, or the collective of microorganisms inhabiting our body, also plays a crucial role in brain function. In recent decades, it has become clear that microbes, especially those living in the gut, influence behavior, emotions, and cognitive processes. This interaction between the brain and microbes is known as the microbiome-gut-brain axis.
Some microbes can affect the levels of neurotransmitters, such as serotonin, which is produced in the gut, and influence inflammatory processes that, in turn, may impact the functioning of the nervous system. For example, disruptions in the balance of the microbiota are associated with the development of depression, anxiety disorders, and even neurodegenerative diseases such as Alzheimer’s disease.
Evolution and Development of These Systems
Over time, through the process of evolution, the systems in various animal species, including humans, became increasingly complex and adapted to the surrounding environment. In the human brain, several levels of development can be distinguished: from ancient structures found in our ancestors, including reptiles, to more complex and specialized regions, such as the neocortex, responsible for abstract thinking, planning, and self-awareness.
In reptiles and their ancestors, including early mammals, there was a part of the brain responsible for basic survival functions, such as instincts, aggression, and sexual behavior. As evolution progressed, and more complex cognitive functions developed, new structures were added to this ancient brain, such as the limbic system, which is responsible for emotions, and the neocortex, which developed in mammals and enables more complex cognitive tasks like abstraction, planning, and self-reflection.
These changes led to the creation of brain structures that process information not only based on current events but also in anticipation of future states, allowing adaptation to the changing conditions of the environment. Brain evolution not only improved survival mechanisms but also created conditions for more complex forms of behavior, such as social interactions, empathy, and language.
Brain Development in Octopuses
The brain of octopuses has a remarkable structure and functional features that distinguish it from the brains of mammals. While octopuses do not possess the same complex brain system as mammals, they demonstrate a high level of cognitive abilities such as learning, tool use, problem-solving, and even signs of personality.
The octopus brain is divided into several parts, with the majority of its mass concentrated in the head. However, two-thirds of its neurons are located in the arms. This unique structure allows each arm to operate relatively independently and make its own decisions. This trait provides octopuses with exceptional flexibility in interacting with their environment and adapting to changing conditions.
Differences in Brain Function Between Octopuses and Humans
Mammals, including humans, developed complex social structures, which contributed to the evolution of a more centrally organized brain. As mammals, we have a highly developed cerebral cortex (especially the frontal lobes), which is responsible for functions such as planning, self-control, and abstract thinking. Our brain is also closely connected to the hypothalamus and the endocrine system, which allows hormones like cortisol and oxytocin to regulate behavior in response to external and internal stimuli.
In contrast, the octopus brain, while also highly developed, functions somewhat differently. The concentration of neurons in their arms allows octopuses to make decisions at a local level without needing to send signals to the central brain. This provides them with remarkable autonomy and the ability to adapt to a variety of situations. For example, octopuses can solve problems related to spatial perception and object manipulation, not only thanks to their central brain but also through their body, which is a unique feature.
In both cases – in mammals and octopuses – the brain serves as an adaptive organ that processes information about the external world and makes decisions based on the organism’s current needs. However, while mammals developed a central brain to coordinate actions and social interactions, octopuses use local brain structures to maintain a high degree of independence for their body parts. This difference reflects distinct evolutionary survival strategies, where mammals rely on collective behavior and complex social interactions, while octopuses depend on individual decision-making and flexibility in manipulating their environment.
The Bayesian Approach to the Mind: The Free Energy Principle and Predictive Coding Theory
Predictive Coding and its foundations, related to Bayesian approaches, play a central role in contemporary understanding of how the brain perceives and processes information. Unlike traditional views of perception, where the brain simply reacts to sensory data, the theory of predictive coding argues that the brain actively constructs models of the world and uses them to predict future events. These predictions are then compared with the actual sensory information received through the senses. Prediction error – the difference between what the brain expects and what it actually perceives – serves as a signal for updating the mental model. This process allows the brain to minimize energy costs, accelerating perception and increasing adaptability, which forms the basis for the effective functioning of cognitive processes.
In recent decades, the theory of predictive coding has increasingly been seen as part of the broader Free Energy Principle, which links it with Bayesian inference, Active Inference, and other approaches focused on minimizing uncertainty and adapting to environmental changes.1. However, despite the growing interest in this integrative approach, predictive coding itself remains a fundamental concept for understanding how the brain constructs models of the world and updates them based on new data. This work will focus primarily on predictive coding, its neurobiological mechanisms, and its role in cognitive processes.
The historical roots of the theory of predictive coding indeed trace back to the works of Pierre-Simon Laplace, who laid the foundation for the concept of determinism. Laplace, one of the first to consider ideas of probability and determinism in the context of predicting the future, proposed that if one had complete knowledge of the current state of the universe, the future could be predicted with absolute certainty. His hypothesis of the “Laplace Demon,” which could predict the future with perfect accuracy, was based on the idea that if we knew all the parameters of microstates, including the position and velocity of every particle, all events – including human thoughts and actions – could be predicted.
This idea of an all-knowing observer and the ability to predict future events based on complete knowledge of present conditions provided an early conceptual foundation for understanding how the brain processes information and makes predictions about the future. Predictive coding and the free energy principle are modern extensions of this concept, where the brain continually updates its internal models of the world to minimize prediction errors and uncertainty.
However, the concept of prediction and world modeling began to develop much later. In the 18th and 19th centuries, Laplace’s ideas about determinism started to be questioned by contemporary philosophers and scientists such as Isaac Newton, Carl Friedrich Gauss, and others. Ideas related to probabilistic calculations and uncertainty gained popularity with the development of statistics and thermodynamics.
The shift toward probabilistic thinking marked a key turning point in the evolution of predictive models. It became increasingly clear that the world is not fully deterministic and that knowledge of the present state is often insufficient to predict the future with absolute certainty. This uncertainty was formally recognized in statistical mechanics, which introduced the concept of entropy – a measure of disorder or uncertainty in a system. As a result, the idea that the brain might work with probabilities, updating predictions based on new information, became more plausible and relevant in the context of cognitive neuroscience.
In the 20th century, the works of Klaus Heisler, Richard Feynman, and Jan Frenkel represented a significant step toward understanding how predictions can operate in conditions of uncertainty and how the brain can construct hypotheses in the context of probability and imperfection. These scientists proposed mathematical approaches that ultimately laid the foundation for the theory of predictive coding in neurobiology.
Equally important contributions to the development of the idea of prediction and coding theory came from researchers in the field of neuroscience in the mid-20th century, such as Benjamin Libet and Nobel laureates Roger Sperry and Jean-Pierre Chevalier. For example, Libet conducted experiments that demonstrated the brain starts the decision-making process several seconds before a person becomes consciously aware of their choice, challenging the idea of full conscious control over behavior.
However, theories similar to predictive coding began to actively develop only in the late 20th and early 21st centuries. A key role in this was played by research into neuroplasticity and the brain’s adaptive mechanisms. Neurobiological studies, including investigations of neurotransmitters such as dopamine and the influence of neural networks, allowed for significant insights into how the brain uses prediction and models to perceive the surrounding world. Founders of predictive coding theory, such as Karl Friedrich von Weizsäcker and Gregory Hooper, proposed that the brain is constantly forming hypotheses about the future based on past experience and correlating them with incoming sensory information.
Bayes’ theorem, proposed by the English mathematician Thomas Bayes in the 18th century, became an important mathematical tool for analyzing and updating probabilistic hypotheses in light of new data.
The essence of the theorem is that it allows for recalculating the probability of a hypothesis based on new data. Bayes’ theorem describes how the belief (or probability) in a hypothesis is updated in response to new information. In the context of the brain, this theorem can be used to explain how neural networks update their predictions about the future, considering both old and new experiences.
In the context of predictive coding theory, this theorem and formula illustrate how the brain updates its hypotheses (or predictions) about the world based on new sensory data. When the brain encounters new events (data), it revises its prior probability (predictions) to incorporate these data, which helps improve the accuracy of future predictions.
Thus, this process reflects a key feature of predictive coding: the brain does not simply react to data, but actively revises its expectations based on new inputs, always striving to minimize prediction errors.
The application of Bayes’ theorem to neurobiology and cognitive science became possible in the 1980s when scientists began to understand how the brain could use probabilistic methods to solve problems of uncertainty. In this paradigm, the brain is seen as a “Bayesian inference” (interpreter) that formulates hypotheses about the world and updates them in response to sensory information using principles of probability. The Bayesian model suggests that the brain maintains probabilistic models of future events and adjusts them based on prediction errors, which is directly connected to the theory of predictive coding.
This updating of probabilistic hypotheses is crucial because it allows the brain not only to adapt to changes in the environment but also to account for uncertainty in the world, even when information is incomplete. In this sense, Bayes’ theorem and its applications have become fundamental to understanding how the brain, when faced with uncertainty, can improve its predictions and forecast the future based on prior knowledge.
Thus, the connection between predictive coding theory and Bayes’ theorem became a key point in the development of neurobiological models explaining how the brain processes information and uses probabilistic computations to predict the future. Bayes’ theory, as the foundation for handling uncertainty and adaptation, provided an important mathematical and cognitive tool for understanding how the brain functions in the context of constant uncertainty and the ever-changing world.
Predictive Coding as an Adaptive Mechanism
The principle behind the theory of predictive coding is that the brain does not simply react to external stimuli, but actively predicts them using existing models of the world. The brain constructs hypotheses about what will happen in the future and compares them with current sensory information. If the predictions match reality, the prediction error is minimized, allowing the brain to use its resources efficiently. If an error occurs – when there is a mismatch between the prediction and reality – the brain updates its models of the world, which helps improve perception and adaptation.
This approach allows the brain to save energy and effort by minimizing the need to process all information from scratch. Instead of interpreting data anew each time, the brain works with simplified models that it constantly updates based on new sensory data. This significantly speeds up information processing and reduces energy expenditure. For example, when a person is walking down the street, their brain does not analyze each step individually but simply uses its predictions about what should happen in the next second.
Predictive Coding operates at different levels, ranging from simple sensory signals (such as sounds or colors) to complex social interactions and abstract ideas. At lower levels, the brain predicts basic sensory signals, such as shapes and movements, while at higher levels, it predicts more complex phenomena, such as people’s intentions or social interaction scenarios.
The Role of Hormones, Neurotransmitters, and Microbiota in Prediction
The effectiveness of predictive coding mechanisms also depends on various external and internal factors. Hormones, neurotransmitters, gut microbiota, and injuries can significantly influence the brain’s ability to predict and adapt.
Cortisol, the stress hormone, can impair the brain’s ability to adjust its predictions. For example, high levels of cortisol may disrupt the process of updating the world model, leading to persistent perceptual errors and increased anxiety. Neurotransmitters such as dopamine play a key role in reward and motivation processes, as well as in strengthening or weakening certain brain predictions. Recent studies have also shown that gut microbiota can influence cognitive functions and even the brain’s predictive abilities, as microbes interact with the central nervous system, affecting our mood and perception.