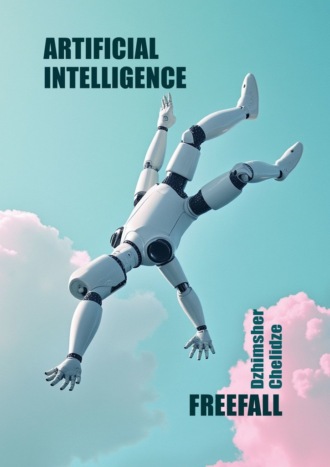
Полная версия
Artificial intelligence. Freefall
However, it is important to understand that zones are not static and can change over time. Therefore, the team must constantly evaluate its context and adapt its approach to retrospectives. The purpose of retrospectives is not to apply Agile indefinitely, but to lead the team from a situation of uncertainty to a situation of complex ordered or simple ordered systems. Only then will retrospectives bring benefits and pleasure to the team and the project”.”
Answer GigaChat: “Agile is a software development methodology that has become very popular in recent years. It offers a flexible approach to project management that allows you to quickly respond to changes and adapt to new requirements. However, the endless application of Agile can lead to problems.
One of the main reasons why Agile is infinitely wrong to apply is that it can lead to a situation of uncertainty. In Agile projects, we often encounter changes in requirements and priorities, which can cause uncertainty about what needs to be done next.
Conducting retrospectives is one way to deal with this uncertainty. Retrospectives help the team understand what was done right and what needs to be improved in the future. They also help the team move from a situation of uncertainty to a situation of complex ordered or simple ordered systems.
However, if we endlessly apply Agile without doing retrospectives, then we may be left in a situation of uncertainty. This can lead to the team spending a lot of time fixing bugs and adapting to new requirements, rather than creating new functionality.
In addition, the endless use of Agile can lead to the fact that the team will constantly work on small tasks and will not have time to create large functional blocks. This may cause the project to develop slowly and not meet the customer’s expectations.
In general, Agile is a great approach to project management, but its endless application can lead to problems. Conducting retrospectives helps the team move from a situation of uncertainty to a situation of complex ordered or simple ordered systems and improve the quality of the team’s work.”
Yandex GPT2’s answer: “The endless use of Agile can lead to incorrect decisions and product quality problems. It is important to understand that Agile is not a universal solution for all projects and teams, and its constant use can lead to loss of efficiency and productivity.
One of the key principles of Agile is to conduct retrospective sessions that help the team analyze their past successes and failures. However, if these retrospectives are held constantly, it can lead the team away from solving real problems and tasks.
Instead of helping the team develop and become more effective, constant retrospectives can lead to a situation where the team is constantly discussing the same problems and cannot move forward. This can create a situation of uncertainty when the team does not know what to do next, or vice versa, it can lead to the creation of complex and disordered systems that only complicate the work process.
To avoid this, it is important to determine when and how often to conduct retrospective sessions. They should focus on analyzing specific issues and opportunities for improvement, rather than discussing the same issues over and over again.
It’s also important to understand that each team and project is unique, and what works for one team may not work for another”.”
YandexGPT 3 Pro: “Why Agile might Agile be the wrong choice?
Agile is a project management methodology that involves an iterative approach to software development. It includes regular team meetings, constant interaction with the customer, and readiness for changes.
However, using Agile is not always the right solution. In some cases, the use of this methodology can lead to negative consequences.
Here are a few reasons why Agile might be the wrong choice:
Not suitable for large projects. Agile is best suited for small projects where you can quickly respond to changes. In large projects that require more careful planning and coordination, Agile can lead to chaos and loss of control.
Not suitable for projects with clear requirements. If the project has clear requirements that are not subject to change, Agile can lead to unnecessary spending of time and resources.
Not suitable for projects with a long development cycle. Agile involves short development cycles that allow you to respond quickly to changes. In projects with a long development cycle, this may cause the project to constantly change and make it impossible to complete.
It is important to understand that Agile is not a universal methodology that is suitable for all projects. The choice of methodology should be based on the specifics of the project, its goals and objectives.
How to avoid negative consequences of using Agile?
To avoid negative consequences of using Agile, it is necessary to carefully analyze the project and determine whether this methodology is suitable for its implementation. If you decide to use Agile, you must follow the following rules:
Conduct regular retrospectives. Retrospectives allow the team to analyze their work and identify weaknesses. This helps you avoid repeating mistakes and improve your work efficiency.
Set clear goals and deadlines. This will help the team focus on completing tasks and avoid wasting time.
Maintain communication with the customer. This will allow you to receive timely feedback and make necessary changes to the project.
Conducting retrospectives should lead away from the situation of uncertainty to the situation of complex ordered or simple ordered systems. This will allow the team to analyze their work, identify weaknesses and take measures to eliminate them.
In conclusion, Agile is an effective methodology that can bring many benefits if applied correctly. However, its use requires careful planning and analysis”.”
AI Search’s response: “Hello, friends! Today I would like to share my thoughts on why Agile, despite its popularity and effectiveness, is often used incorrectly. I will pay particular attention to why conducting retrospectives is an important tool for moving from a situation of uncertainty to orderly or complex systems.
Agile is a philosophy and approach to software development that changes the traditional approach to project management, making it more flexible and adaptive to change. However, despite its obvious advantages, Agile can be applied incorrectly, and as a result, its potential is not fully revealed.
One of the main problems of using Agile incorrectly is the lack of regular retrospectives. A retrospective, as an expert assessment of past work, helps teams take into account the changes, shortcomings, and improvements that have occurred when planning future iterations. This allows you to hone the work process and increase efficiency. However, some teams often ignore conducting retrospectives or conduct them unsystematically.
By not conducting regular retrospectives, teams miss opportunities to see their issues, validate or change their vision, and customize development milestones. As a result, teams may be stuck in a situation of uncertainty and unable to move forward.
One of the main principles of Agile is to ensure a fast response to changes and continuous improvement of the work process. If retrospectives are not conducted regularly, teams are not sufficiently aware of their mistakes and omissions, which makes it difficult to progress towards achieving order and efficiency.
In addition, conducting regular retrospectives helps teams move from a situation of uncertainty to complex or simple ordered systems. Starting with simple, streamlined systems where rules and processes are easily defined and controlled, teams can gradually move to more complex systems where rules are more ambiguous and require more adaptation.
So, flashbacks are an important part of the Agile process and help teams move from uncertainty to order and complex systems. They allow teams to recognize their mistakes and shortcomings, as well as see areas for improvement. Do not neglect regular retrospectives so that your team can maximize the potential of Agile and achieve high performance.
I wish you successful work in applying Agile and continuous improvement of your processes! Be flexible and persistent and considerate”.”
Well, and my post: “Why is Agile not eternal and not a panacea?”
“In recent years, everyone declares that they are Agile.
Let’s be honest: most people hide banal chaos under this. After all, Agile is not about the lack of planning. In addition, he is very sensitive to the implementation of certain rules and to the stability of the team, its motivation. If you don’t meet these conditions, don’t use Agile in the future.
And using the same Scrum, Kanban or other approaches should lead to the absence of the need to implement Agile projects.
But why?
Let’s remember that Agile was originally designed to work and implement projects in a highly uncertain environment.
There is even a special tool – model Kenevin, that helps you understand what situation you are in and what approach you need to choose, what to focus on. So, in ordered systems (simple or complex situations), Agile, on the contrary, is contraindicated, because it reduces the cost of achieving results. That is, Agile is effective in cases where you need to do – I don’t know what”. But it’s not about efficiency.
Now let’s take a look at the retrospective. All approaches within Agile involve regular retrospectives, analysis of their work, and interaction with the client / customer / partner. In other words, the very logic of these tools is to get away from an uncertain situation and learn how to predict them and become more effective.
If you constantly (every six-months or a year) change jobs or constantly launch new products, and do not replicate certain solutions (which is strange for a business), then yes, you need to be Agile.
But if you have a segment in the AC, and you have worked with experience and expertise on typical approaches / products that need to be adjusted and adapted in a small part, then sooner or later you must move away from Agile and come to an ordered system where cascading or hybrid approaches are needed. These retrospectives should lead you to understand what customers want in 90%of cases and how the organization works.
As a result, if you are Agile on an ongoing basis and everywhere, and not for the period of rebuilding / launching / adapting, then this may indicate that:
– you are not following the Agile tools;
– you haven’t found your product or niche, and you haven’t developed the necessary expertise;
– you always have a unique product / project (which should be reflected in the high price of your services);
– the organization is “sick” from the inside, and this way you mask high turnover, lack of work on processes, etc.
What do you think about it?”
The quality of neural network responses is more like a weak student who just picks up similar words, but doesn’t understand what he’s talking about. Could I get a similar post from the neural network? So that she can convey my meaning? Yes, of course, but I wrote my post in 25 minutes, and how long would it take me to try to achieve such a result from AI? And does it look like a really thinking intelligence? And in general, on Intelligence?
The fact is that our thinking is strongly tied to ideas and images. That is, basically in thinking we use an approach from the general to the particular, we think in images, and then from some vague, but understandable to us at the unconscious level of abstractions we come to the smallest details. In parallel, we conduct a bunch of associations, completely tied again to images. It is not for nothing that there are so many metaphors, comparisons, allusions and word changes in human speech.
Modern neural networks “think” quite differently. They simply do not know the concepts of “meaning”, “essence”, “idea”, as well as the process of reflection itself. The texts generated by the neural network look logical not because the neural network really understands what it is writing about, but because it is able to calculate which words are most often next to each other in the texts available for its training on a given topic. Pure mathematics based on calculating probabilities.
However, for the sake of justice, we should also note significant progress in this direction. Now developers are trying to teach AI to think: it asks itself questions and, giving answers, draws conclusions. This is an imitation of an internal dialog to improve the quality of content, which is typical for models from Open AI at the end of 2024 and Yandex GPT 4 Pro RC.
Here is an example of the response of the Yandex GPT 4 Pro RC model to the request” Write a post about why Agile is infinitely applied incorrectly. Emphasize that conducting retrospectives should lead away from the situation of uncertainty to the situation of complex ordered or simple ordered systems.”
Yandex GPT 4 Pro RC Response: “Why Agile doesn’t Always Work: how retrospectives can help move from uncertainty to order.
Agile is a flexible approach to project management that allows you to quickly adapt to changes and improve processes based on feedback. However, despite all the advantages, applying Agile without considering the context can lead to problems. In this post, we will look at why Agile should not be applied indefinitely and how retrospectives help to move from a situation of uncertainty to ordered systems.
Problems of infinite Agile Application
1. Lack of clear structure. Agile is based on the principles of flexibility and adaptability, which can lead to a lack of a clear structure and action plan. This can make it difficult to make decisions and manage risks.
2. Complexity of evaluating results. Without a clear plan and criteria for evaluating results, it is difficult to determine how well the project is progressing. This may raise doubts about the effectiveness of the approach.
3. Risk of losing control. The endless use of Agile can lead to a loss of control over the project. Without clear boundaries and constraints, the team may lose focus and start to deviate from the original goal.
4. Inefficient use of resources. If the team is constantly adapting to new conditions, this can lead to inefficient use of resources. Instead of focusing on key tasks, the team will spend time making constant changes.
5. Reduced team motivation. Constant adaptation and changes can cause stress and fatigue for the team. This can reduce motivation and productivity.
How retrospectives help you move to streamlined systems
Retrospectives are one of the key Agile tools that help the team analyze their work and identify areas for improvement. They allow us to move from a situation of uncertainty to more ordered systems, where processes and decisions become more predictable and manageable.
Here are some ways that flashbacks can help:
– Identifying problems and their solutions. Retrospectives allow you to identify problems that have arisen in the course of work, and find ways to solve them. This helps you avoid making mistakes again in the future.
– Improve processes. Retrospectives help you understand which processes are working well and which need to be improved. This allows you to optimize your work processes and increase efficiency.
– Increase motivation. When a team sees the results of their efforts and understands that they bring benefits, it increases motivation and job satisfaction.
– Create a culture of continuous improvement. Retrospectives foster a culture in which continuous improvement and adaptation are the norm. This helps the team to be more flexible and ready for changes.
In general, retrospectives are an important tool for moving from a situation of uncertainty to more structured and orderly processes. They help the team adapt to changes, improve their processes, and achieve their goals”.”
Yes, the model has become academic and it is clear that these are excerpts from articles, and not reflections. But this is a huge progress, and you can already work with the model and use it as a tool.
However, with only weak AI available and dreaming of a more or less strong one, various researchers already distinguish super-strong AI (ASI, Artificial Superintelligence) in their classification.
This is about an AI, that:
– It can solve both routine and creative tasks;
– instantly navigates uncertainty even without a network or internet connection;
– adapts problem solving to the context of circumstances and available opportunities / resources;
– understands people’s emotions (not only through a text query, but also based on the analysis of facial expressions, voice timbre, and other parameters) and takes them into account in your work;
– able to interact independently with the real world to solve problems.
This is the kind of AI that we only see in science fiction movies so far. Even AI itself writes about ASI as a “hypothetical concept” and “a subject of science fiction and active research in the field of artificial intelligence” (YandexGPT 3 Pro). This is a certain desired point in the distant future, which is not yet possible to reach.
Chat GPT 4 describes ASI as follows: “hypothetical artificial intelligence system with intelligence superior to human. This is not just an advanced AI, but a system that can adapt, learn and develop independently, exceeding human abilities in learning, problem solving, cognitive functions and self-improvement.
Super-strong AI, or ASI, will be able to understand and process many types of data (text, images, sound, video), which will allow it to perform verbal tasks and make decisions. It will use advanced AI technologies such as multidimensional language models (LLMs), multi-bit neural networks, and evolutionary algorithms.
Currently, ASI remains a conceptual and speculative stage in the development of AI, but it represents a significant step forward from the current level of AI”.”
And if there are hundreds of weak AIS now, for each task, then there will be only dozens of strong AIS (most likely there will be a division in directions, we will consider this in the next block), and the super-strong AI will be one for the state and even the entire planet.
Limitations on the path to strong AI
To be honest, I have little faith in the rapid emergence of a strong or super-strong AI.
First, this is a very costly and complex task from the point of view of regulatory restrictions. The era of uncontrolled AI development is ending. More and more restrictions will be imposed on it. We’ll discuss AI regulation in a separate chapter.
The key trend is a risk-based approach. So, in a risk-based approach, strong and super -strong AI will be at the upper level of risk. This means, that legislative measures will also be protective.
Secondly, this is a difficult task from a technical point of view, and a strong AI will be very vulnerable.”
Now, in the mid-2020s, creating and training a strong AI requires huge computing power. So, according to Leopold Aschenbrenner, a former Open AI employee from the Super alignment team, it will require the creation of a data-center worth a trillion US dollars. And its power consumption will exceed all current electricity generation in the United States.
We also need complex AI models (orders of magnitude more complex than the current ones) and a combination of them (not just LLM for query analysis). In other words, it is possible to exponentially increase the number of neurons, build connections between neurons, and coordinate the work of various segments.
At the same time, it should be understood that if human neurons can be in several states, and activation can occur “in different ways” (biologists will forgive me for such simplifications), then machine AI is a simplified model that does not know how to do this. Simply put, machine’s 80—100 billion neurons are not equal to a human’s 80—100 billion. The machine will need more neurons to perform similar tasks. The same GPT4 is estimated at 100 trillion parameters (conditionally neurons), and it is still inferior to humans.
All this leads to several factors.
The first factor is that increasing complexity always leads to reliability problems, and the number of failure points increases.
Complex AI models are difficult to both create and maintain from degradation over time, in the process of operation. AI models need to be constantly “serviced”. If this is not done, then a strong AI will begin to degrade, and neural connections will be destroyed, this is a normal process. Any complex neural network, if it is not constantly developing, begins to destroy unnecessary connections. At the same time, maintaining relationships between neurons is a very expensive task. AI will always optimize and search for the most efficient solution to the problem, which means, that it will start turning off unnecessary energy consumers.
That is, the AI will look like an old man with dementia, and the “life” period will be greatly reduced. Imagine what a strong AI with its capabilities can do, but which will suffer from memory loss and sharp reversals to the state of the child? Even for current AI solutions, this is an actual problem.
Let’s give a couple of simple real-life examples.
You can compare building a strong AI to training your human muscles. When we first start working out in the gym and get involved in strength training, bodybuilding, then progress is fast, but the further we go, the lower the efficiency and increase in results. You need more and more resources (time, exercise, and energy from food) to progress. Yes, even just holding the form is becoming more and more difficult. Plus, the increase in strength comes from the thickness of the muscle section, but the mass grows from the volume. As a result, the muscle will at some point become so heavy that it will not be able to move itself, and may even damage itself.
Another example of the complexity of creating, but already from the field of engineering, is Formula 1 races. For example, a 1-second lag can be eliminated if you invest 1 million and 1 year. But to win back the crucial 0.2 seconds, it may already take 10 million and 2 years of work. And the fundamental limitations of the design of the car can force you to reconsider the whole concept of a racing car.
And even if you look at ordinary cars, everything is exactly the same. Modern cars are more expensive to create and maintain, and without special equipment, it is impossible to change even a light bulb. If you take modern hyper cars, then after each departure, entire teams of technicians are required for maintenance.
If you look at it from the point of view of AI development, there are two key parameters in this area:
– number of layers of neurons (depth of the AI model).
– the number of neurons in each layer (layer width).
Depth determines how great the AI’s ability to abstract is. Insufficient depth of the model leads to a problem with the inability to perform deep system analysis, superficiality of this analysis and judgments.
The width of the layers determines the number of parameters / criteria that the neural network can use on each layer. The more they are, the more complex models are used and the more complete reflection of the real world is possible.
However, if the number of o layers has a linear effect on the function, then the width does not. As a result, we get the same analogy with muscle – the size of top AI models (LLM) exceeds a trillion parameters, but models 2 orders of magnitude smaller do not have a critical drop in performance and quality of responses. More important is what data the model is trained on and whether it has a specialization.
Below are statistics for LLM models from different manufacturers.
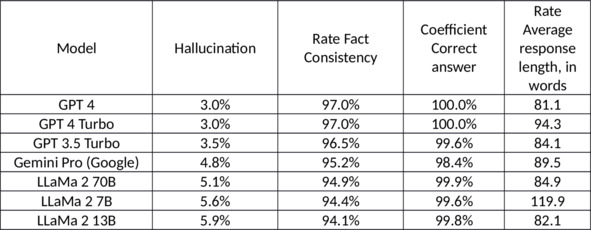
Compare the indicators LLaMa 2 70B, LLaMa 2 7B, LLaMa 2 13B. Indicators 70B, 7B and 13B conditionally demonstrate the complexity and training of models – the higher the value, the better. But as we can see, the quality of responses does not radically change, while the price and labor costs for development increase significantly.