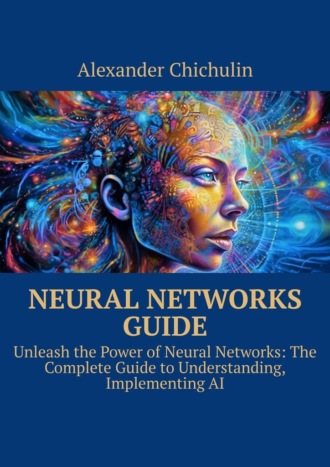
Полная версия
Neural networks guide. Unleash the power of Neural Networks: the complete guide to understanding, Implementing AI
5. Dimensionality Reduction:
– Dimensionality reduction techniques reduce the number of input features while retaining important information.
– Principal Component Analysis (PCA) and t-SNE (t-Distributed Stochastic Neighbor Embedding) are popular techniques for dimensionality reduction.
– Dimensionality reduction can help mitigate the curse of dimensionality and improve training efficiency.
6. Train-Test Split and Cross-Validation:
– To evaluate the performance of a neural network, it is essential to split the data into training and testing sets.
– The training set is used to train the network, while the testing set is used to assess its performance on unseen data.
– Cross-validation is another technique where the dataset is divided into multiple subsets (folds) to train and test the network iteratively, obtaining a more reliable estimate of its performance.
These data preprocessing techniques are applied to ensure that the data is in a suitable form for training neural networks. By cleaning the data, handling missing values, scaling features, and reducing dimensionality, we can improve the network’s performance, increase its efficiency, and achieve better generalization on unseen data.
Handling Missing Data
Missing data is a common challenge in datasets and can significantly impact the performance and reliability of neural networks. In this chapter, we will explore various techniques for handling missing data effectively:
1. Removal of Missing Data:
– One straightforward approach is to remove instances or features that contain missing values.
– If only a small portion of the data has missing values, removing those instances or features may not significantly affect the overall dataset.
– However, this approach should be used cautiously as it may result in loss of valuable information, especially if the missing data is not random.
2. Mean/Median Imputation:
– Mean or median imputation involves replacing missing values with the mean or median value of the respective feature.
– This technique assumes that the missing values are missing at random (MAR) and the non-missing values carry the same statistical properties.
– Imputation helps to preserve the sample size and maintain the distribution of the feature, but it can introduce bias if the missingness is not random.
3. Regression Imputation:
– Regression imputation involves predicting missing values using regression models.
– A regression model is trained on the non-missing values, and then the model is used to predict the missing values.
– This technique captures the relationships between the missing feature and other features, allowing for more accurate imputation.
– However, it assumes that the missingness of the feature can be reasonably predicted by other variables.
4. Multiple Imputation:
– Multiple imputation is a technique where missing values are imputed multiple times to create multiple complete datasets.
– Each dataset is imputed with different plausible values based on the observed data and their uncertainty.
– The neural network is then trained on each imputed dataset, and the results are combined to obtain more robust predictions.
– Multiple imputation accounts for the uncertainty in imputing missing values and can lead to more reliable results.
5. Dedicated Neural Network Architectures:
– There are specific neural network architectures designed to handle missing data directly.
– For example, the Masked Autoencoder for Distribution Estimation (MADE) and the Denoising Autoencoder (DAE) can handle missing values during training and inference.
– These architectures learn to reconstruct missing values based on the available information and can provide improved performance on datasets with missing data.
The choice of handling missing data technique depends on the nature and extent of missingness, the assumptions about the missing data mechanism, and the characteristics of the dataset. It is important to carefully consider the implications of each technique and select the one that best aligns with the specific requirements and limitations of the dataset at hand.
Dealing with Categorical Variables
Categorical variables pose unique challenges in neural networks because they require appropriate representation and encoding to be effectively utilized. In this chapter, we will explore techniques for dealing with categorical variables in neural networks:
1. Label Encoding:
– Label encoding assigns a unique numerical label to each category in a categorical variable.
– Each category is mapped to an integer value, allowing neural networks to process the data.
– However, label encoding may introduce an ordinal relationship between categories that doesn’t exist, potentially leading to incorrect interpretations.
2. One-Hot Encoding:
– One-hot encoding is a popular technique for representing categorical variables in a neural network.
– Each category is transformed into a binary vector, where each element represents the presence or absence of a particular category.
– One-hot encoding ensures that each category is equally represented and removes any implied ordinal relationships.
– It enables the neural network to treat each category as a separate feature.
3. Embedding:
– Embedding is a technique that learns a low-dimensional representation of categorical variables in a neural network.
– It maps each category to a dense vector of continuous values, with similar categories having vectors closer in the embedding space.
– Embedding is particularly useful when dealing with high-dimensional categorical variables or when the relationships between categories are important for the task.
– Neural networks can learn the embeddings during the training process, capturing meaningful representations of the categorical data.
4. Entity Embeddings:
– Entity embeddings are a specialized form of embedding that takes advantage of the relationships between categories.
– For example, in recommendation systems, entity embeddings can represent user and item categories in a joint embedding space.
– Entity embeddings enable the neural network to learn relationships and interactions between different categories, enhancing its predictive power.
5. Feature Hashing:
– Feature hashing, or the hashing trick, is a technique that converts categorical variables into a fixed-length vector representation.
– It applies a hash function to the categories, mapping them to a predefined number of dimensions.
– Feature hashing can be useful when the number of categories is large and encoding them individually becomes impractical.
The choice of technique for dealing with categorical variables depends on the nature of the data, the number of categories, and the relationships between categories. One-hot encoding and embedding are commonly used techniques, with embedding being particularly powerful when capturing complex category interactions. Careful consideration of the appropriate encoding technique ensures that categorical variables are properly represented and can contribute meaningfully to the neural network’s predictions.
Part II: Building and Training Neural Networks
Feedforward Neural Networks
Structure and Working Principles
Understanding the structure and working principles of neural networks is crucial for effectively utilizing them. In this chapter, we will explore the key components and working principles of neural networks:
1. Neurons:
– Neurons are the basic building blocks of neural networks.
– They receive input signals, perform computations, and produce output signals.
– Each neuron applies a linear transformation to the input, followed by a non-linear activation function to introduce non-linearity.
2. Layers:
– Neural networks are composed of multiple layers of interconnected neurons.
– The input layer receives the input data, the output layer produces the final predictions, and there can be one or more hidden layers in between.
– Hidden layers enable the network to learn complex representations of the data by extracting relevant features.
3. Weights and Biases:
– Each connection between neurons in a neural network is associated with a weight.
– Weights determine the strength of the connection and control the impact of one neuron’s output on another’s input.
– Biases are additional parameters associated with each neuron, allowing them to introduce a shift or offset in the computation.
4. Activation Functions:
– Activation functions introduce non-linearity to the computations of neurons.
– They determine whether a neuron should be activated or not based on its input.
– Common activation functions include sigmoid, tanh, ReLU (Rectified Linear Unit), and softmax.
5. Feedforward Propagation:
– Feedforward propagation is the process of passing the input data through the network’s layers to generate predictions.
– Each layer performs computations based on the inputs received from the previous layer, applying weights, biases, and activation functions.
– The outputs of one layer serve as inputs to the next layer, progressing through the network until the final predictions are produced.
6. Backpropagation:
– Backpropagation is an algorithm used to train neural networks.
– It calculates the gradients of the loss function with respect to the network’s weights and biases.
– Gradients indicate the direction and magnitude of the steepest descent, guiding the network’s parameter updates to minimize the loss.
– Backpropagation propagates the gradients backward through the network, layer by layer, using the chain rule of calculus.
7. Training and Optimization:
– Training a neural network involves iteratively adjusting its weights and biases to minimize the difference between predicted and actual outputs.
– Optimization algorithms, such as gradient descent, are used to update the parameters based on the calculated gradients.
– Training typically involves feeding the network with labeled training data, comparing the predictions with the true labels, and updating the parameters accordingly.
Understanding the structure and working principles of neural networks helps in designing and training effective models. By adjusting the architecture, activation functions, and training process, neural networks can learn complex relationships and make accurate predictions across various tasks.
Implementing a Feedforward Neural Network
Implementing a feedforward neural network involves translating the concepts and principles into a practical code implementation. In this chapter, we will explore the steps to implement a basic feedforward neural network:
1. Define the Network Architecture:
– Determine the number of layers and the number of neurons in each layer.
– Decide on the activation functions to be used in each layer.
– Define the input and output dimensions based on the problem at hand.
2. Initialize the Parameters:
– Initialize the weights and biases for each neuron in the network.
– Random initialization is commonly used to break symmetry and avoid getting stuck in local minima.
3. Implement the Feedforward Propagation:
– Pass the input data through the network’s layers, one layer at a time.
– For each layer, compute the weighted sum of inputs and apply the activation function to produce the layer’s output.
– Forward propagation continues until the output layer is reached, generating the network’s predictions.
4. Define the Loss Function:
– Choose an appropriate loss function that measures the discrepancy between the predicted outputs and the true labels.
– Common loss functions include mean squared error (MSE) for regression problems and cross-entropy loss for classification problems.
5. Implement Backpropagation:
– Calculate the gradients of the loss function with respect to the network’s weights and biases.
– Propagate the gradients backward through the network, layer by layer, using the chain rule of calculus.
– Update the weights and biases using an optimization algorithm, such as gradient descent, based on the calculated gradients.
6. Train the Network:
– Iterate through the training data, feeding it to the network, performing forward propagation, calculating the loss, and updating the parameters through backpropagation.
– Adjust the learning rate, which controls the step size of parameter updates, to balance convergence speed and stability.
– Monitor the training progress by evaluating the loss on a separate validation set.
7. Evaluate the Network:
– Once the network is trained, evaluate its performance on unseen data.
– Use the forward propagation to generate predictions for the evaluation dataset.
– Calculate relevant metrics, such as accuracy, precision, recall, or mean squared error, depending on the problem type.
8. Iterate and Fine-tune:
– Experiment with different network architectures, activation functions, and optimization parameters to improve performance.
– Fine-tune the model by adjusting hyperparameters, such as learning rate, batch size, and regularization techniques like dropout or L2 regularization.
Implementing a feedforward neural network involves translating the mathematical concepts into code using a programming language and a deep learning framework like TensorFlow or PyTorch. By following the steps outlined above and experimenting with different configurations, you can train and utilize neural networks for a variety of tasks.
Fine-tuning the Model
Fine-tuning a neural network involves optimizing its performance by adjusting various aspects of the model. In this chapter, we will explore techniques for fine-tuning a neural network:
1. Hyperparameter Tuning:
– Hyperparameters are settings that determine the behavior of the neural network but are not learned from the data.
– Examples of hyperparameters include learning rate, batch size, number of hidden layers, number of neurons in each layer, regularization parameters, and activation functions.
– Fine-tuning involves systematically varying these hyperparameters and evaluating the network’s performance to find the optimal configuration.
2. Learning Rate Scheduling:
– The learning rate controls the step size in parameter updates during training.
– Choosing an appropriate learning rate is crucial for convergence and preventing overshooting or getting stuck in local minima.
– Learning rate scheduling techniques, such as reducing the learning rate over time or using adaptive methods like Adam or RMSprop, can help fine-tune the model’s performance.
Конец ознакомительного фрагмента.
Текст предоставлен ООО «ЛитРес».
Прочитайте эту книгу целиком, купив полную легальную версию на ЛитРес.
Безопасно оплатить книгу можно банковской картой Visa, MasterCard, Maestro, со счета мобильного телефона, с платежного терминала, в салоне МТС или Связной, через PayPal, WebMoney, Яндекс.Деньги, QIWI Кошелек, бонусными картами или другим удобным Вам способом.